Taking the Pain out of Personalized Marketing
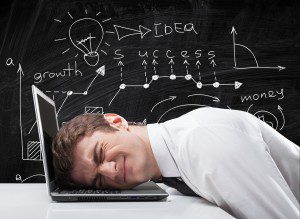
Stress. Young businessman with his head squeezed between a laptop keyboard and a rock
Predictive analytics improves marketing results. That’s hard to dispute these days, but it’s not quite right.
Relevant messaging and attractive offers improve marketing results. Predictive analytics has the “potential” to help.
It’s only potential because there is so much involved in doing it right. There is a broad diversity of algorithms and approaches for all sorts of purposes. As a result, most companies enlist skilled data specialists and find themselves clarifying their objectives because the typical approach is going to be expensive.
But it does not have to be anymore, depending on what you want to do.
How personalized marketing gets complicated
Most predictive technologies are based on the principle of finding customer attributes or behaviors that reliably predict outcomes. For example, what is it about a given customer that seems to indicate a likely interest in buying Product A?
For this approach to work best, you want to gather and evaluate as many customer attributes and behaviors as possible because you never know what might have predictive value. After all, the idea that data science can do a better job at this than you is what got you here in the first place.
So your data scientists will start asking for information. It may be a little at first, but it grows in the quest for greater accuracy. Demographics, CRM data, website click streams, social media interactions, maybe real-time location data — maybe data you have to buy from a third party.
They want to build up extensive customer profiles, a “360-degree view” of each customer. There’s always more data that “could” be useful. That’s the promise of Big Data after all, right? How long before they start asking to use increasingly sensitive personal data, too?
Next thing you know, your predictive analytics project is being fed by a much larger and voracious data sourcing, acquisition, cleansing, enrichment, modeling, integration, storage, governance and ongoing maintenance project involving lots of people and time.
When you finally have a complete set of data in the right place and format, it’s time to start the predictive modeling, usually the rarified domain of statisticians and analytics specialists — today’s data scientists. The job is to derive formulas and rules that “score” or group customers, based on their attributes, against your objective (knowing who is most likely to buy Product A).
There are various techniques and a lot of software to select from or combine, and a lot of testing is needed to verify and refine accuracy. Then if you also want to know who might be interested in Product B, it’s a whole new exercise. If you have hundreds or thousands of products, you’ll have to decide which ones are worth this effort — hopefully you get it right.
Personalized marketing made a whole lot easier
What if you can’t afford all this time or cost, just to get going?
Fortunately, there are new technologies that squeeze the most predictive power from relatively little information. For example, it is possible to accurately predict the products each of your customers is most likely interested to buy from just a few, noncontroversial and readily available fields of transaction data.
This is the opposite of the typical approach. This new approach makes its predictions from a much narrower range of information but requires lots of transaction history. The advantages include the potential to automate the analytics and, more importantly, eliminate the need for expensive data preparation projects because the few data fields needed can easily be extracted from the order management system or data warehouse.
What if you don’t have the necessary skills or cannot afford all the software, assuming you can figure out what to buy?
Again, you might be in luck, depending on what you want to do. By constraining the problem domain to specific objectives, a new breed of solutions automates the analytics. Following the previous example, no one has to do any data modeling to predict the products every customer is most interested to buy. If packaged as an easy-to-access software service, marketers can get the predictive metrics they need by themselves. No special analytic skills required.
Be careful, however. Every generation of analytics vendors since at least the 1980s has claimed theirs is finally the solution for line-of-business workers without data analytics skills.
Predictive analytics is advanced data science. The only real way to make it accessible to anyone is to automate it, so no one has to do it, and package it as an easy-to-access service. That may only work in constrained circumstances, but the cost-effectiveness can be tremendous.
How to get practical results marketers can use
Ultimately, working with data is tough. It has to be interpreted and its imperfections accommodated. In the end, a predictive modeling exercise produces “insights” — insights about who your customers are, their intent or what they might be interested in, usually associated with a score or probability.
It’s useful stuff, but you still have to translate these insights into action. How will you market to a customer whose loyalty score is, say, 8.6?
One thing that helps is to define and refine a complete process for specific, actionable purposes. A process or service purposed to deliver an online recommendation engine or generate mailing lists with personalized offers leaves little doubt about how to leverage the results from your predictive analytics. In other words, the goal is not so much the scores, but an actionable deliverable.
Predictive analytics always used to require people or close-knit teams skilled in the necessary data science and the tools to practice it. The process also needed people who understand the full meaning, relationships and flaws in all the data, as well as the business context, the company’s marketing objectives, and knowledge of how best to apply it all to your marketing programs. That’s a team that can solve a lot of problems given enough time.
But if you cannot afford that, or want to take a few problems off their plate, simpler solutions are emerging that are just as effective for certain specific problems. For example, if you just want to know what products to offer each customer in your personalized email campaign, there are quicker, simpler ways get that than ever before.
That makes it accessible to a lot more marketers.