Overcoming Obstacles To Relevant Marketing
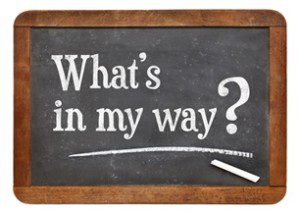
What is in my way?
There are several different names for relevant marketing: personalized marketing, individualized marketing, one-to-one marketing, micro marketing are some we’ve heard. Lots of companies want to do it, primarily for two reasons: customers are objecting to “one size fits all” spam, and vendors believe that relevance raises response rates. But whatever you call it, and regardless of your motivation, there is little consensus on how to communicate in a meaningful and unique way to each of your customers.
Sending each customer a communication that is relevant to her sounds like a formidable task. Most marketers quake at the thought of sending a million unique emails to a million unique customers. It’s not the sophistication of the ESP that’s a roadblock. Many ESPs can handle variable data and some can’t, so companies that want individualized communications find the vendors who can.
It’s not the “last mile,” either. Preparing the file needed by your ESP with the appropriately organized and formatted data can be a difficult task, but it’s not rocket science. Some marketing service providers have automated the task and can do it in a very few minutes.
What’s The Real Showstopper?
The component that seems like the showstopper is creating a million or more unique messages for your customers. Short of ten million monkeys hammering away at typewriters (in between writing the plays of Shakespeare), how do you get unique messages at scale? Even if you have rich demographic data, it’s effectively impossible to generate a million appropriate and unique messages. Ten are possible, but even 50 are a stretch. Segments and profiles are not going to solve this problem.
The answer is surprisingly simple, but not immediately obvious: You don’t need a million unique messages. A million unique product offers will do the job of individualizing your emails just fine, thank you.
Generating a million offers
If you are a retailer, eCommerce vendor or catalog marketer, you probably have thousands of SKUs. If you are using an email template with slots for, say, four or five products, there are many permutations of four or five products for those template slots. Good customer analytics can deliver purchase probabilities for those slots.
The probabilities can be for up-sells (categories from which a customer has already purchased), or for cross-sells (products not yet purchased). If you are an eCommerce vendor, I guarantee you have a lot of one-time buyers for whom those cross-sells are tempting. There are now packaged solutions for coming up with millions of relatively different product combinations, each combination tailored to a particular customer.
If you have a few million customers and a hundred thousand SKUs, you can generate the individualized purchase probabilities for all of your customers in less than a day. Fifty thousand customers and a smaller product set take less than an hour. If you have the customers, products, transaction data and the smarts to analyze them properly, creating individualized offers for millions of customers is a standard, business-as-usual procedure. Individualized marketing is the way to utilize those analytics.
How powerful is your microscope?
Individualizing through offers rather than messages was a not-obvious leap that took marketing services companies a long while to discover. The methodology to get those individualized offers is taking even longer to gain acceptance, and in retrospect should have been much more obvious: more granular analytics.
Most customer analytics today is done at the segment level: look at all the customers in a given Zip code, or all the customers who bought product X. The standard technique is regression analysis, where the object is to find a single mean value that fits all the customers in the segment.
The overlooked, alternative is to do the analysis at the individual customer level. If you want to know what an individual customer is going to buy, you need to look at what that customer has purchased, not at the behavior of a group of customers like your targeted customer. You don’t want the mean value for a group; you want the individual prediction for particular customer.
Why RFM Doesn’t Work Here
Analyzing at the individual customer level means rethinking the mathematical approach. Techniques like RFM (Recency-Frequency-Monetary value) are inappropriate because they ignore product information. How are you going to predict what an individual customer is likely to buy if your analysis ignores what that customer has previously purchased?
In hindsight, this seems so obvious. Probably the low-hanging fruit captured by RFM was valuable enough to let marketers ignore more powerful methods. Today, however, customers are no longer willing to accept being carpet-bombed by spam and they are demanding more relevant email communications. Happily the tools and techniques are finally available to meet their demands.
Piece originally featured in Email Insider.